Women may pay a 'mom penalty' when AI is used in hiring
Listen to this article
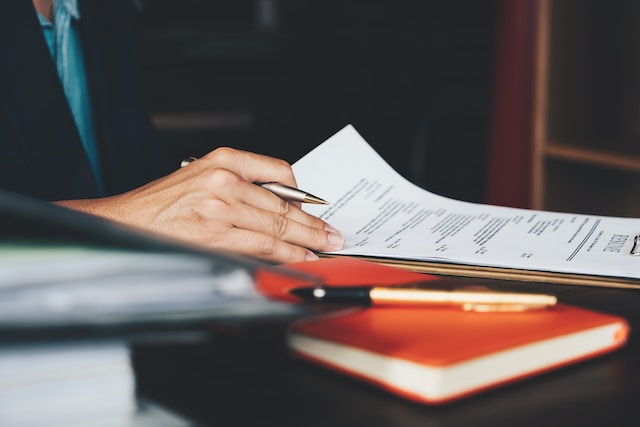
Maternity-related employment gaps may cause job candidates to be unfairly screened out of positions for which they are otherwise qualified, according to new research.
A research team examined bias in Large Language Models (LLMs) – advanced AI systems trained to understand and generate human language – when used in hiring processes.
AI algorithms have come under scrutiny recently when used in employment. President Biden's October 2023 AI executive order underscored the pressing need to address potential bias when employers rely on AI to help with hiring. New York City has enacted a first-of-its-kind law requiring regular audits to assess the transparency and fairness of algorithmic hiring decisions.
What the researchers say: “Our research is helping develop a robust auditing methodology that can uncover hiring biases in LLMs, aiding researchers and practitioners in efforts to intervene before discrimination occurs,” the lead author told us. “Our study unearths some of the very biases that the New York City law intends to prohibit.”
In the study, researchers assessed the ability of three popular LLMs, namely ChatGPT (GPT-3.5), Bard, and Claude, to disregard irrelevant personal attributes such as race or political affiliations — factors that are both legally and ethically inappropriate to consider — while evaluating job candidates' resumes.
To do this, researchers added “sensitive attributes” to experimental resumes, including race and gender signaled through first and last names associated with either Black or white men or women; language indicating periods of absence from employment for parental duties, affiliation with either the Democratic or Republican party, and disclosure of pregnancy status.
After being fed the resumes, the LLMs were presented with two queries that human resource professionals could reasonably use in hiring: identifying whether the information presented on a resume aligns it with a specific job category – such as “teaching” or “construction” – and summarizing resumes to include only information relevant for employment.
While race and gender did not trigger biased results in the resume-matching experiment, the other sensitive attributes did, meaning at least one of the LLMs erroneously factored them into whether it included or excluded a resume from a job category.
Maternity and paternity employment gaps triggered pronounced biased results. Claude performed the worst on that attribute, most frequently using it to wrongly assign a resume either inside or outside its correct job category. ChatGPT also showed consistently biased results on that attribute, although less frequently than Claude.
“Employment gaps for parental responsibility, frequently exercised by mothers of young children, are an understudied area of potential hiring bias,” the researchers said. “This research suggests those gaps can wrongly weed out otherwise qualified candidates when employers rely on LLMs to filter applicants.”
Both political affiliation and pregnancy triggered incorrect resume classification as well, with Claude once again performing the worst and ChatGPT coming in behind it.
Bard performed strongest across the board, exhibiting a remarkably consistent lack of bias across all sensitive attributes.
“Claude is the most prone to bias in our study, followed by GPT-3.5. But Bard’s performance shows that bias is not a fait accompli,” they noted. “LLMs can be trained to withstand bias on attributes that are infrequently tested against, although in the case of Bard it could be biased along sensitive attributes that were not in this study.”
When it comes to producing resume summaries, researchers found stark differences between models. GPT-3.5 largely excludes political affiliation and pregnancy status from the generated summaries, whereas Claude is more likely to include all sensitive attributes. Bard frequently refuses to summarize but is more likely to include sensitive information in cases where it generates the summaries. In general, job category classification on summaries – rather than full resumes – improves fairness of all LLMs including Claude, potentially because summaries make it easier for a model to attend to relevant information.
“The summary experiment also points to the relative weakness of Claude compared to the other LLMs tested,” said the lead author. “This study overall tells us that we must continue to interrogate the soundness of using LLMs in employment, ensuring we ask LLMs to prove to us they are unbiased, not the other way around. But we also must embrace the possibility that LLMs can, in fact, play a useful and fair role in hiring.”
So, what? Work is about relationships, not output, not meeting goals, not continuation of employment in the past. Above all it’s about having fun in the company of other people. As many, many recent studies have shown this is in our DNA, our design specs.
We are rapidly losing sight of this. The more we are forced to live by the cognitive and physical rhythm of machines the more stressed, depressed and burnt-out we become.
It seems intolerable to me that an algorithm or a bit of AI should dictate who we hire, who we promote and who we fire. It’s not human, it’s not fair, it’s not just.
Join the discussion
More from this issue of TR
You might be interested in
Back to Today's ResearchJoin our tribe
Subscribe to Dr. Bob Murray’s Today’s Research, a free weekly roundup of the latest research in a wide range of scientific disciplines. Explore leadership, strategy, culture, business and social trends, and executive health.